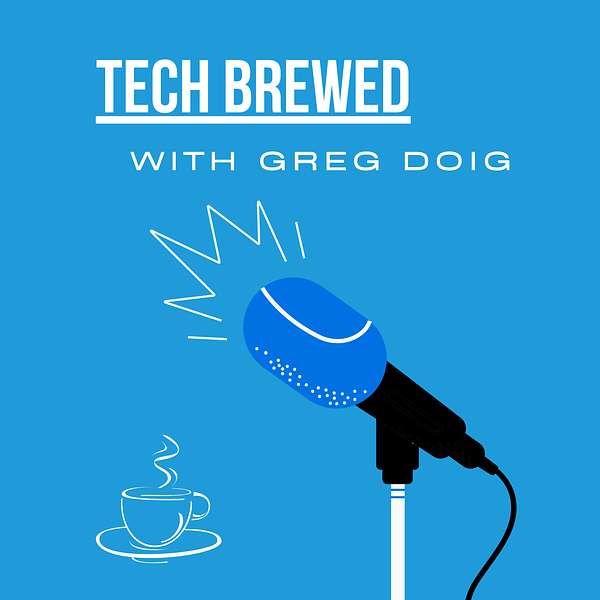
Tech Brewed
Welcome to Tech Brewed, your go-to podcast for the latest in technology products, training, and tips. Whether you're a tech enthusiast or a business professional, our show offers valuable insights into the ever-evolving world of technology.
What We Cover
Home and Business Technology Products
We delve into the latest gadgets and tools that can enhance your home and business environments. From smart home devices to enterprise-level solutions, we keep you updated on the best products for your needs.
Technology Training and Tips
Our episodes are packed with practical advice and training tips to help you maximize your tech investments. Whether you want to improve your cybersecurity or optimize your workflow, we've got you covered.
Creative Technology Software and AI
In today's digital age, creative technology software and artificial intelligence are game-changers. We explore how these innovations transform various industries and offer insights into their practical applications.
Featured Segments
AI and Its Impact
Discover how AI is changing the workplace and driving innovation. Our discussions range from improving audio quality with AI to extending life through advanced technologies.
Practical AI
Our goal is to make artificial intelligence accessible and practical for everyone. We break down complex topics into easy-to-understand segments, ensuring you stay ahead of the curve.
Join us on Tech Brewed for a deep dive into the tech world, where we blend expert knowledge with practical advice to help you navigate the digital landscape. Subscribe now and stay informed on the latest trends and innovations!
Tech Brewed
Discover the Power of RAG: AI’s Smart Librarian
Today we're diving deep into the fascinating universe of retrieval augmented generation, abbreviated RPG. If you've been following advancements in artificial intelligence and machine learning, you've likely heard of this transformative approach to enhancing A.I. capabilities. So go ahead, grab a coffee, tea, your drink of choice, and let's brew up some tech knowledge. Today is all about retrieval augmented generation, a technique revolutionizing how A.I. interacts with vast pools of data. Whether you're in research, development, or just curious about cutting edge tech, Wragg offers some incredible applications.
Subscribe to the weekly tech newsletter at https://gregdoig.com
Hey there, tech enthusiasts. Welcome to another sip of tech knowledge on Tech Brewed, your go to spot for all things tech. So grab your favorite mug, and let's dive in together. Cheers to learning something new. Today, we're diving deep into the fascinating universe of retrieval augmented generation, abbreviated r a g. If you've been following advancements in artificial intelligence and machine learning, you've likely heard of this transformative approach to enhancing AI capabilities. So go ahead, grab a coffee, tea, or drink of choice, and let's brew up some tech knowledge. Today is all about retrieval augmented generation, a technique revolutionizing how AI interacts with vast pools of data. Whether you're in research, development, or just curious about cutting edge tech, RAG offers some incredible applications. Let me break it down for you. At its core, RAG enhances AI by combining retrieval and generation. Think of it as a smart librarian that doesn't just retrieve the right book for your question, but also summarizes and explains it in a way you'll understand. This means faster answers, more relevant information, and smarter systems. So what makes RAGS so special? Imagine a scenario where your AI assistant doesn't just generate answers based on pre trained data. Instead, it actively retrieves the most up to date relevant documents from sources like relational databases, vector databases, or even the web. Then it uses advanced natural language processing to craft an answer tailored to your query. Here's the exciting part, the RAG workflow involves several advanced components each playing a crucial role in making this process seamless and efficient. Let's walk through them. Number 1, query construction. This is where it all begins. The system takes your question and transforms it into a structured query. Advanced tools like Text TO SQL or Text to Cypher convert natural language into a format that databases can understand. There's also something called a self query retriever, which automatically generates metadata filters to make the search even more precise. Number 2. Query translation. Now, not every question is straightforward. What if your question is ambiguous? That's where query decomposition comes in. It rephrases or breaks down your input to retrieve the most relevant results. Imagine asking, what's the best coffee machine for under$200? The system might interpret it as fine coffee machines and filter by price under $200. Smart. Right? Number 3. Routing. Not all data lives in the same place. The RAG system intelligently routes your query to the correct data source. Whether that's a relational database, a graph database, or a vector store filled with embeddings. Think of it as directing traffic on the data superhighway. Number 4, retrieval. Once the query lands in the right place the retrieval mechanism takes over. It filters results based on relevance ranking them with advanced models like RAG Fusion or even rank GPT. Here's the kicker, if the retrieve documents don't fully answer your question the system goes back for another round, a process known as active retrieval. And number 5. Indexing and generation. Finally, all this data isn't useful unless it's organized and presented effectively. That's where indexing and generation come in. Techniques like semantic splitting or hierarchical indexing ensure that data is easy to navigate. On the generation side, the AI can synthesize its findings into a concise and informative response. And now this may sound complex, and it is. But the applications are game changing. From building intelligent customer support systems to creating more intuitive research tools, RAG is opening doors we never thought possible. So why does RAG matter? In a world drowning in data, RAG is a lifeline. It makes AI smarter, more efficient, and more capable of solving real world problems. Whether you're a developer, a tech enthusiast, or just someone who loves learning about what's next, this is a technology you'll want to keep on your radar. That's it for today's episode. I hope you enjoyed this deep dive into retrieval augmented generation. If you did, be sure to subscribe to the podcast, share the episode, and as always, you can sign up for the newsletter at gregdoyg.com. Thanks for tuning in, and as always, keep brewing those ideas. And that's a wrap. Thanks for sticking around, friends. I really appreciate your time and hope you found our time together enjoyable. Don't forget to like, share, or comment if you feel inclined. Until next time, take care and keep smiling.